Understanding Synthetic Data

Joe Scharf
2 min read
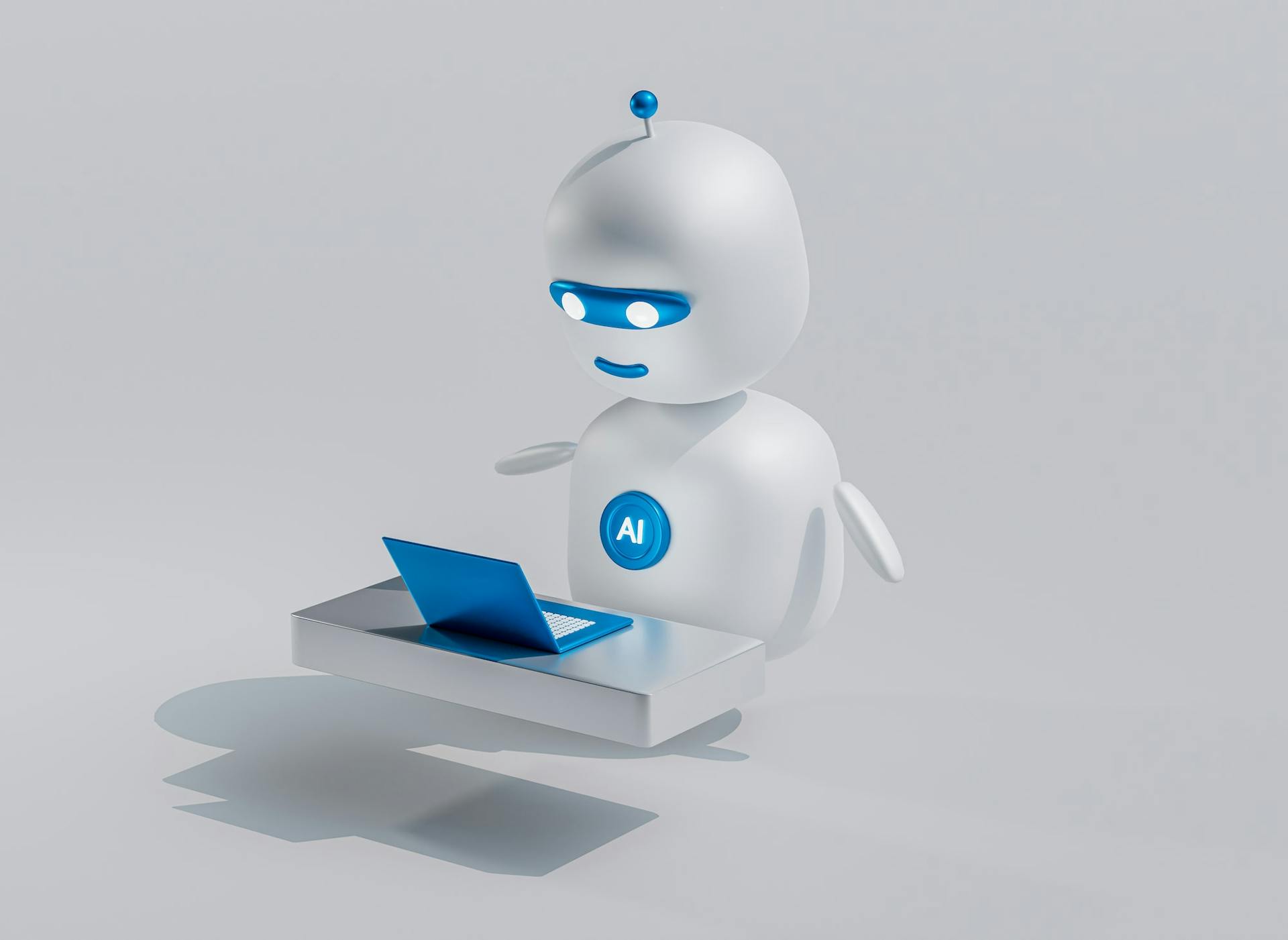
Photo by Mohamed Nohassi / Unsplash
Synthetic data refers to data that's artificially created rather than generated by actual events. There is a growing need to harness real-world production data for application development, machine learning modeling, and generative AI training. There are many issues concerning data privacy that make it difficult to create sanitized production data sets for use in various development or research sectors. Gartner estimates that by 2024, 60% of data for AI applications will be synthetic.
Here are a few key elements involved in synthetic data technology:
Generation Techniques: There are various methods to create synthetic data, such as rule-based methods, deep learning techniques, and generative models like Generative Adversarial Networks (GANs). The choice of technique depends on the complexity of the dataset and the type of data (numeric, categorical, time-series, text, etc.).
Maintaining Data Utility: One of the challenges in creating synthetic data is to maintain the statistical properties of the original dataset. The synthetic data should have the same or similar distribution, correlations, and variability as the original data to be useful in testing or training models.
Privacy Protection: One of the main uses of synthetic data is to protect privacy. The process involves creating new data that mimic the properties of the original data but do not contain any actual sensitive or personal information. The synthetic data should be generated such that it's impossible (or extremely hard) to reverse-engineer it to find the original data.
Validation: After creating synthetic data, it's important to validate it to ensure it accurately represents the original data's properties. This could involve statistical tests, or using the synthetic data to train a model and then comparing the performance of that model to one trained on the original data.
In the case of DBSnapper, we use synthetic data technology to replace sensitive information in database snapshots. This allows teams to work with data that maintains the structure and variability of the original data while ensuring full compliance with data privacy regulations.
Would you like to learn more about our synthetic data capabilites? Drop us a line at contact@dbsnapper.com.